The Role of AI in Mental Health
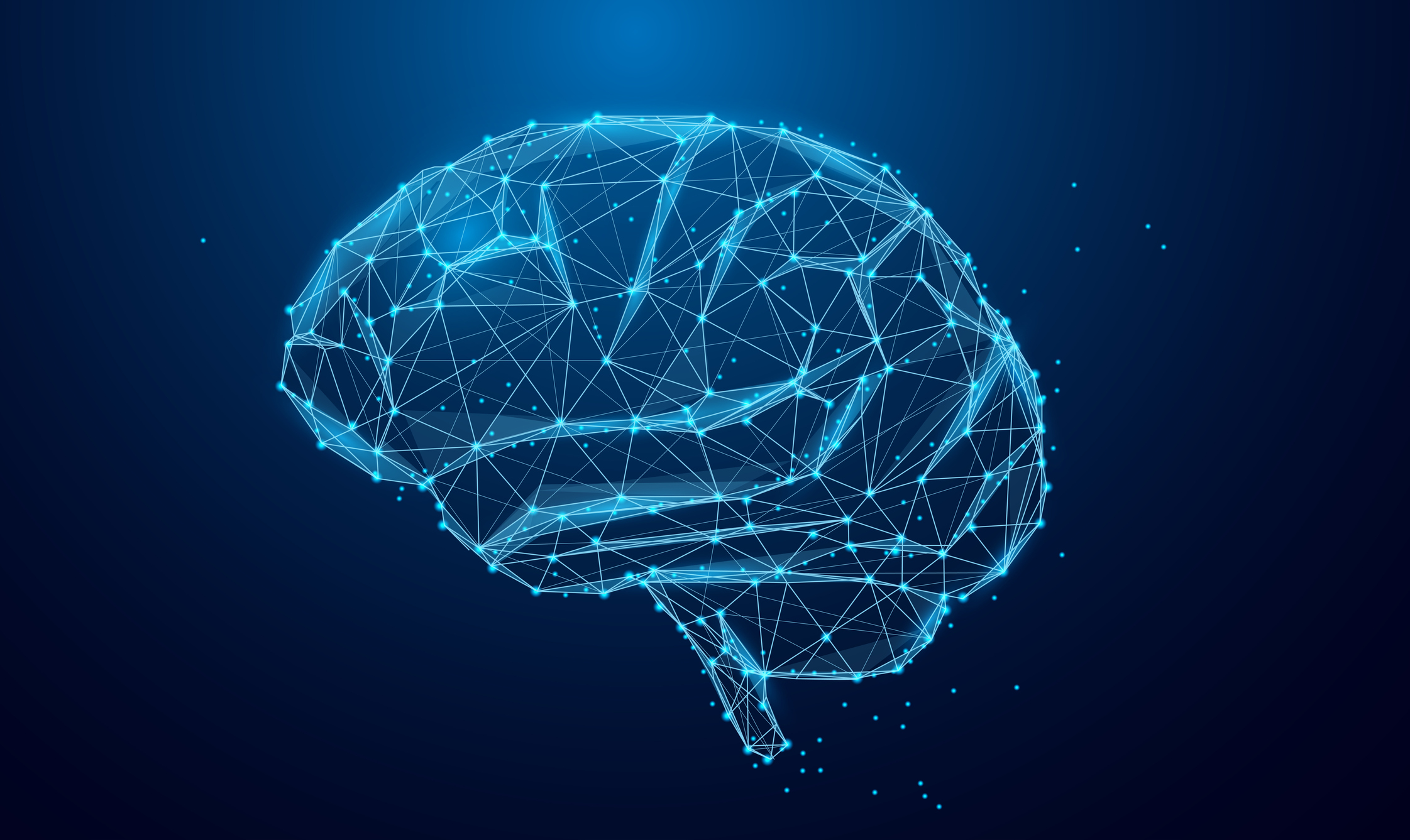
Summary
Artificial Intelligence (AI) holds the promise of transforming virtually any industry, including healthcare. Doctors are already using systems that leverage AI to help them make better decisions and automate tasks, yet this is just the beginning. Over time, consumers and end users will see the benefits of these algorithms — and those benefits will extend over time to the fields of healthcare, medicine, and mental health.
Artificial Intelligence (AI) holds the promise of transforming virtually any industry. AI is advancing due to many factors such as well-studied algorithms, availability of cost-effective processing systems, and accessibility of technology stacks to the public. There’s also a lot more data available to be processed and used for training purposes. The roles of AI in industries are novel and can be disruptive. For example:
- AI has transformed how financial institutions manage the risk of investments, and decisions to provide credit to someone or not.
- AI has transformed manufacturing by predicting when mechanical parts may fail or predicting forecasts based on ever-changing global environments.
- AI has transformed eCommerce by predicting customer purchasing decisions and understanding the customer intent by analyzing a multitude of data about the customer.
- AI has improved how WiFi networks can be explained/managed, for example, by predicting what would be the result of the Speed Test if a device on the network performed this test, at any given time.
The examples of roles of AI in your favorite industry are numerous and growing.
Healthcare is not immune to these disruptions. Doctors are using systems that leverage AI to help them make better decisions, automate some of the error-prone and mundane tasks that they (or their support staff) perform otherwise. For example, some systems transcribe doctor-patient meetings and automatically attach that transcript and actions as part of the patient visit record.
Healthcare areas such as mental health haven’t seen concrete adoption of AI — yet. One possible reason may be that it’s somewhat difficult to measure the accuracy of the output of AI algorithms. The above examples of AI in other industries can be measured for accuracy in the following ways:
- The accuracy of an AI system to assess the risk of extending credit to someone can be measured by whether the person defaulted on the credit or not (binary)
- The accuracy of an AI system to predict mechanical part failures can be measured by whether the part fails after some time or not (binary)
- The accuracy of an AI system to predict whether a customer will purchase a product or not can be measured by the purchase decision (binary)
- Transcription software accuracy can be measured by someone who reads the transcription and listens to the recording (binary)
- The accuracy of speed test prediction can be measured against an actual speed test
With mental health, it’s a bit more difficult as many of the factors in mental health are subjective. For example, it can be difficult to measure an individual’s depression or anxiety levels. The standard practice is to ask a series of questions of the patient where the answers come back as a ranked scale. The interpretations of those questions can vary between individuals, and the answers can vary if asked several times of the same person in quick succession.
To illustrate this point, consider one of the questions from the PHQ-9 survey, a standard 9-question survey asked from the patients to determine their depression levels.
Over the last two weeks, how often have you been bothered by “feeling little interest or pleasure in doing things”?
Answer choices: Not at all, Several days, More than half the days, Nearly every day
There is a lot that can be left to interpretation when answering these questions, which makes it difficult for an AI system to work with. In this particular case, the overall depression of a patient is calculated by some formula (e.g. summation) that looks at individual answers to the 9 questions.
All is not lost, however. Many AI systems show promise, and research is both ongoing and expanding all the time in terms of scope.
“As we develop algorithms and systems to fine-tune and optimize pattern matching and predictions of what can happen next given a certain environment, we will gain more confidence in these algorithms.”
Predicting Anxiety and Depression
If the questions asked in the standard surveys are not concrete and answers can vary, how can an AI system better predict the answers?
One approach is to come up with one or several external signals to predict the Overall Depression score or Overall Anxiety score. For example, the patient is asked to type a sentence or speak a sentence, and the AI system tries to predict the overall score by analyzing the speed of typing, or error rate, or tone of speech, including level, accuracy, and clarity. Another similar approach tries to detect the patient’s level of attention by analyzing eye movement or body gestures. Yet another approach asks the patient to perform some (game-like) activities such as picking objects in a list in some order (e.g. numbered objects) and analyzing the accuracy and speed of completion.
As you may guess, these approaches can be difficult to implement and correlate with actual anxiety or depression levels since these levels are somewhat subjective to begin with.
Another approach is to take each question in the standard survey and design an activity or system that is more tailored to the content of the question. For example, for the question on “Having trouble falling or staying asleep, or sleeping too much?” the system can look at the sleep patterns of the patient and try to come up with an answer based on the sleep data. This is a good approach, but some of the questions don’t have an obvious signal that an AI system can interpret. For instance, “Feeling down, depressed, or hopeless?” is just as hard to predict as the overall depression score.
Several companies have tried these approaches, but given that there aren’t any standard or proven implementations of these systems, it can be interpreted that we are at the early stages of adoption of AI in mental health and that there are still a lot of questions that we need to answer.
Effects of Drugs
There are hundreds of drugs that are used to treat one or more aspects of mental health. Many of these drugs are prescription-based, and their use is controlled. However, doctors prescribing these medications sometimes end up prescribing a combination of drugs that aim to balance the side effects of one medication by using another medication. For example, bupropion (antidepressant) drugs may be prescribed in combination with antipsychotic meds (e.g. Abilify), and anti-nausea drugs (because nausea is a side effect of bupropion). How does the combination of these drugs affect depression or anxiety, or alter the sleep patterns or any of several areas that affect the mood and general mental health of a person?
While pharmaceutical companies have done studies as part of their trials of each drug, individual reactions can vary and AI can help patients and doctors in understanding individual reactions and their correlation to other health areas of the patients. Furthermore, AI systems can help identify potential effects from drug combinations.
Predicting Addiction
Addiction to drugs or medications is a huge problem in the U.S. While there are ways for people to receive support in dealing with addiction, including recovery centers, individual and group therapy sessions, it’s extremely easy to relapse. Experiencing relapse can be demotivating and put the patient at an even greater disadvantage in overcoming addiction. It can be helpful if the patient has the tools to know what activities or experiences are likely to cause relapse, or what activities can help them stave off relapse (e.g. more exercise, a different diet, more sleep). AI can help in predicting these cause-and-effect scenarios and provide an individualized view. These systems can also alert the treating doctors and clinicians to reach out to the individual at the time of highest risk of relapse and provide the necessary support and help.
Empowering Caregivers
We often associate AI with replacing decisions made by humans (e.g. self-driving cars). Replacing humans may make sense for some use cases, and it may indeed happen for those cases in the distant future. But on the path to get there AI will help augment human decision-making, not replace it.
One of the fundamental concepts of AI is that the system needs a way to know if a decision by the models proves correct or not. This feedback mechanism is important to fine-tune the model and parameters of the AI algorithms. The quicker and more frequent this feedback mechanism occurs, the faster the algorithm can improve. This feedback mechanism can come in many ways, but a natural way is for this feedback to come from humans themselves. You can see this when your AI-driven photo management system asks you to verify the people in photos that the AI system guessed. You can see this process in action in Apple’s iPhoto, Google Photos, Shutterfly photos, and many similar applications.
In the realm of mental health, AI systems can empower therapists by providing them with suggestions that are the result of analyzing patient data from multiple sources. For example, the AI system can monitor the patient’s tone of voice or body movement and, in combination with the patient’s medication history, sleep patterns or movement patterns, the AI system can suggest the mental state of the patient to the therapist. It can make suggestions of what topics therapists should discuss or avoid discussing to reduce anxiety or depression and generally improve the patient’s mental health. While the AI system is making these suggestions, the therapist can provide feedback to the system, indicating whether the suggestion is valid or not.
Another example of empowering caregivers can be in the form of automating administrative tasks such as generating notes, taking and transcribing notes, generating billing and CPT codes, automating communication with patients as well as other doctors and facilities involved in the patient’s care. The more of these administrative tasks can be automated, the less the caregivers will spend time on them, and the more quality time they will spend with their patients and caring for them.
Conclusion
The field of AI and ML is relatively young and still growing. As we develop algorithms and systems to fine-tune and optimize pattern matching and predictions of what can happen next given a certain environment, we will gain more confidence in these algorithms. The field of applied AI is where consumers and end users will see the benefits of these algorithms — and those benefits will extend over time to the fields of healthcare, medicine, and mental health. It’s an exciting time to be experiencing the growth of the technologies that will empower us as consumers by giving us greater control and visibility.
These solutions may appear to be easy to understand and obvious once it’s explained or observed. Many of these solutions will prove to be very difficult to implement from a technology perspective. It will take some time to fine-tune these systems and train them so their predictions and usage become second nature and trustworthy. Gaining the trust of these predictions will be even tougher for health-related areas. After all, identifying a person incorrectly in your photos has a different consequence than suggesting that a patient take a particular medicine or worse yet, having the system call 911 on a patient’s behalf because some catastrophic outcome is predicted.
Learn More
Get in touch with us.
Contact us at [email protected]